AI in Upstream Oil & Gas - something's missing!
- Jeff Jacobsen
- Jun 15, 2020
- 3 min read
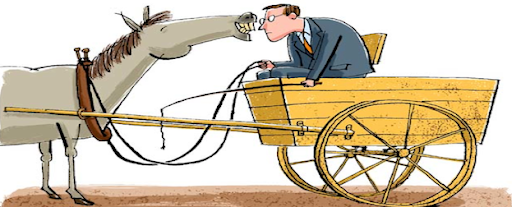
One of my favorite character actors, Jerry Stiller, recently passed away at the age of 92, having given us many, many years of laughter. His iconic role as Frank Costanza helped make Seinfeld, in my opinion, the best sitcom of all time. The scene that illustrates Stiller’s brilliance is his monologue about the hen, the rooster, and the chicken, where he couldn’t understand the sexual relationship between the three. I’ll spare you the dialogue, but it ended with him saying “something’s missing here”. And that is exactly how I feel about AI in general, and more specifically, in the upstream sector of oil and gas.
For starters, what exactly is artificial intelligence, and how may it benefit the upstream sector of the oil and gas industry? From a very simplistic point of view, the artificial in AI represents machines, and as such, AI represents machine intelligence, in contrast to the natural intelligence of human beings. The most basic form of machine learning is predictive analytics, or the ability for a machine to predict outcomes based on historical information.
Predictive analytics is a basic form of statistical analysis, based on the law of large numbers, which states that the more inputs you have, the closer you will be able to predict an outcome. The example most often used is the probability of flipping a coin and have it land heads up. As there are only two options (heads or tails), the probability of landing heads up is 1 out of 2, or 50%. However, if you flip the coin 10 times, it may land heads up 7 times, or 70%. The law states that as you increase the number of flips, you will get closer and closer to 50%. The law of large numbers plays a big role in the use of predictive analytics in the upstream oil and gas industry, as the nature of the data involved in the analytics is neither voluminous nor readily available. And this, my friends, is the crux of the matter – the cart has ended up before the horse.
The cart in this case is the knee-jerk reaction employed when confronting a predictive analytics problem – the design of a model, collection of domain variables, building and testing an algorithm to solve the problem. With the tests completed, it’s time to put the algorithm into practice with real data, i.e. historical data in numbers large enough to satisfy the law of large numbers. And this is where the defecation hits the rotary oscillator – all the work put in by the data scientists is for naught, as the project did not include any data engineering. It would be similar to building a state-of-the-art gathering system, only to find out that there are no pipelines to deliver the product.
The upstream sector of the oil and gas industry must take modest steps forward in the AI arena, realizing the inherent risks in having scarce amounts of poor quality data. I have spent the last 15 years working “in the weeds”, toiling away to create high quality data in large quantities in an automated system. One such system receives PDFs from vendors, sends them to a PDF scraping engine, resulting in data being scraped from the PDF and stored in a database – all in an automated fashion. Imagine the power of this? The ability to create a pipeline of data to serve the algorithms the data scientists spent some much time and effort building.
If your company is stuck due to the lack of consistent, historical data, please reach out to the experts at Blue Ocean Existence (www.BlueOceanExistence.com)
Comments